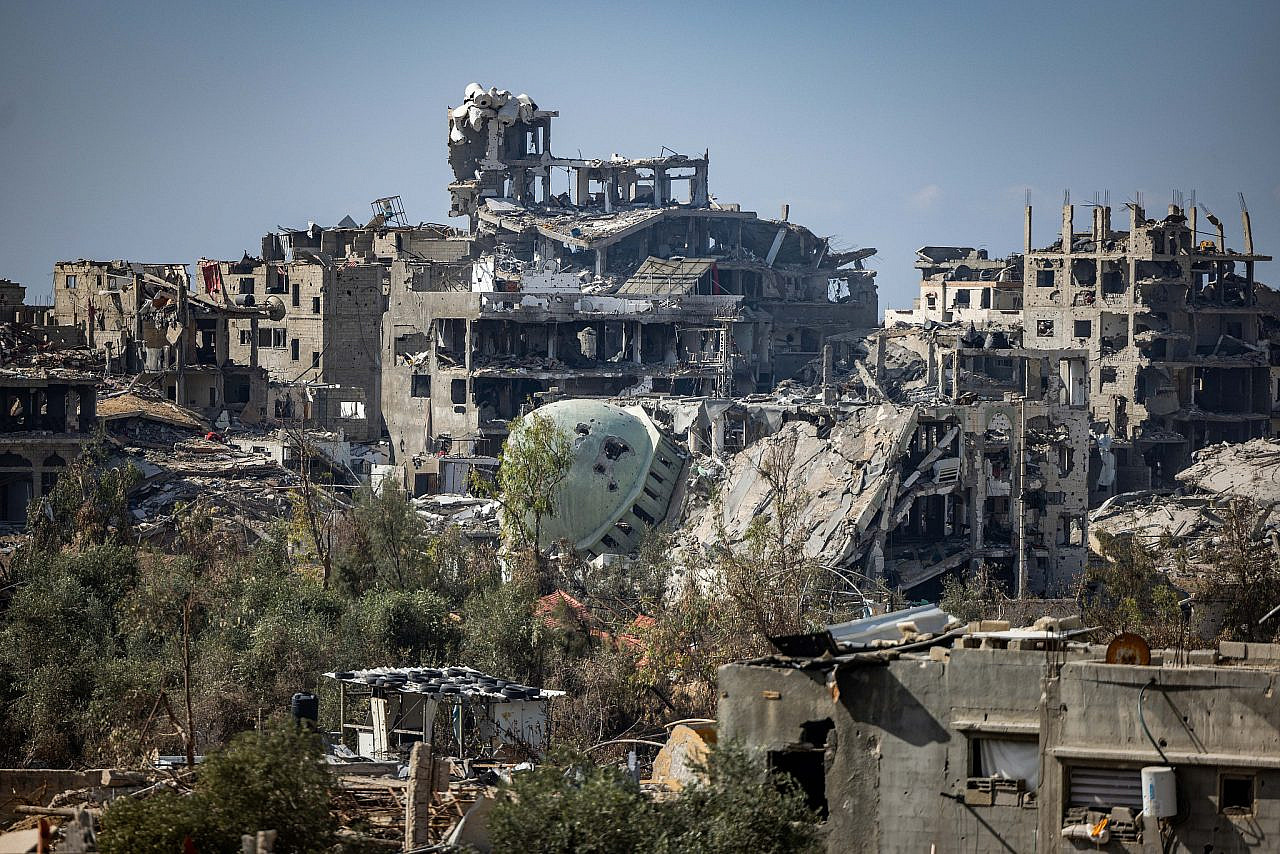
On 11 January 2024, the International Court of Justice opened proceedings on charges of genocide brought by South Africa against Israel’s operations in Gaza. Israel, on its side, frames its military operations as self defense and a justifiable response to the massacre of Israeli civilians by Hamas on 7 October 2023. In the media coverage on Israeli operations in Gaza, one investigative report stood out for those of us who have been following developments in the algorithmic intensification* of military killing machines, a story of Israel’s AI-enabled targeting system named Habsora, or the Gospel.
Headlined ‘“A mass assassination factory”: Inside Israel’s calculated bombing of Gaza,’ the report draws on sources within the Israeli intelligence community who confirm that Israeli Defense Force (IDF) operations in the Gaza strip combine more permissive authorization for the bombing of non-military targets with a loosening of constraints regarding expected civilian casualties. This policy sanctions the bombing of densely populated civilian areas, including high-rise residential and public buildings designated as so called ‘power targets’. Official legal guidelines require that selected buildings must house a legitimate military target and be empty at the time of their destruction; the latter has resulted in the IDF’s issuance of a constant and changing succession of unfeasible evacuation orders to those trapped in diminishingly small areas of Gaza. These targeting practices are presumably facilitated by the extent and intensity of the surveillance infrastructure in the Occupied Palestinian Territories (see Anthony Lowenstein’s The Palestine Laboratory). Moreover, once Israel declares the entire surface of Gaza as a cover for Hamas tunnels, all of which are assumed to be legitimate military targets, the entire strip becomes fair game for destruction.
A direct corollary of this operational strategy is the need for an unbroken stream of candidate targets. To meet this requirement, Habsora is designed to accelerate the generation of targets from surveillance data, creating what one former intelligence officer (quoted in the story’s headline) describes as a “mass assassination factory”. Most notably, the Israeli bombardment of Gaza has shifted the argument for AI-enabled targeting from claims to greater precision and accuracy, to the objective of accelerating the rate of destruction. IDF spokesperson R Adm Daniel Hagari has acknowledged that in the bombing of Gaza “the emphasis is on damage and not on accuracy.” For those who have been advancing precision and accuracy as the high moral ground of data-driven targeting, this admission must surely be disruptive. It shifts the narrative from a technology in aid of adherence to International Humanitarian Law and the Geneva Conventions, to automation in the name of industrial scale productivity in target generation, enabling greater speed and efficiency in killing. As the intelligence sources acknowledge, moreover, Israel’s operations are not indiscriminate but are deliberately designed to create ‘shock’ among the civilian population, under the premise that this will somehow contribute to Israel’s aim of eliminating Hamas.
Israel’s mobilization of algorithmic intensification to accelerate target production should be understood within a wider technopolitical context of so-called network-centric warfare. A project dating back to the 1990s, with roots in the cybernetic imaginary of the Cold War, data-driven warfighting promises a technological solution to the longstanding problem of ‘situational awareness’ as a prerequisite for the perpetuation of military logics. As National Defense Magazine observes of the various proposals for networked warfare “what all these concepts have in common is the vision of a truly networked battlefield in which data moves at the speed of light to connect not only sensors to shooters, but also the totality of deployed forces and platforms.” Data here are naturalised, treated as self-evident signs emitted by an objectively existing world ‘out there,’ rather than as the product of an extensively engineered chain of translation from machine readable signals to ad hoc systems of classification and interpretation. And contra the idea that it is the demonstrated value of data that leads to surveillance and data gathering, data-driven operations are mandated by investment in those infrastructures. The on-faith investment in surveillance and data gathering, in other words, feeds a desire to rely on data for decision-making, however questionable the provenance and chains of inference.
All of this occurs in a context of Israel’s economic commitment to establishing itself as a leading purveyor of high-tech military technoscience, not least in so-called AI-enabled warfighting. For both Ukraine and Israel these wars are an opportunity to boost their arms sales. Battle-tested systems are easier to sell, and US venture capital firms like Eric Schmidt’s Innovation Endeavors, and companies like Palantir, are lining up to be part of the booming weapons industry. Yet enormous questions remain regarding the validity of the assumptions built into these systems about who comprises an imminent threat and about the legitimacy of their targeting functions under the Geneva Conventions and the laws of war. We know that these platforms require continually updated datasets sourced from satellite imagery, drone footage and other surveillance data monitoring the movements and behaviour patterns of individuals and groups, including cell phone tracking, social media, and intercepted communications. But we don’t know how data quality is validated or what assumptions are built into categories like “military objects” or “persons of interest” and their designation as legitimate targets. The evidence from Gaza, whereas of this writing civilian casualties have surpassed 25,000 (and are likely significantly higher), including over 10,000 children and destruction of roughly 70% of Gaza’s buildings and critical infrastructure, the gospel of AI-enabled precision and accuracy has now been revealed as a pretext for the acceleration of unrestrained and criminal acts of killing. While the masters of war enjoy their short-term profits through the promise of technological solutions, critical voices, including a growing number inside Israel, agree that only an immediate ceasefire and unconditional release of hostages can re-open the path to a political solution. Let us hope that the IJC reaches the same conclusion.
*An alternative reading of ‘AI’ suggested by Andrew Clement (personal communication)